Polynomial fitting using Pytorch
Here is a short tutorial on how to fit polynomials using pytorch.
Here is a short tutorial on how to fit polynomials using pytorch.
NOTE: This was written a few years ago. I found this notebook burried in my SSD yesterday and thought I should share it.
Fitting Polynomials
Creating a polynomial module of second order a+bx+cx^2=0
import torch
device = torch.device('cuda' if torch.cuda.is_available() else 'cpu')
class Polynomial_2(torch.nn.Module):
def __init__(self) -> None:
super().__init__()
self.a = torch.nn.Parameter(torch.randn(()))
self.b = torch.nn.Parameter(torch.randn(()))
self.c = torch.nn.Parameter(torch.randn(()))
def forward(self, x):
return self.a+self.b*x+self.c*torch.pow(x, 2)
Generate Parabolic data
import numpy as np
def generate_parabolic_data(N,a,b,c):
"""Genrates parabolic data around a curve a+bx+cx^2=0
Args:
N (int): num_samples
a (float): coeff 1
b (float): coeff 2
c (float): coeff 3
Return: X, Y in the shape (N,)
"""
x = np.linspace(-10, 10, N)
y = a+b*x+c*x**2
return x.astype(np.float32),y.astype(np.float32)
X, Y = generate_parabolic_data(10,1,2,3)
Training loop
num_rows = 1000
lr=1.e-1
num_epochs = 25
batch_size = 16
num_batches = num_rows//batch_size
X, Y = generate_parabolic_data(num_rows,1,2,3)
X = torch.from_numpy(X).to(device)
Y = torch.from_numpy(Y).to(device)
polynomial_model = Polynomial_2()
polynomial_model.to(device)
# Add parameters as a list
optim = torch.optim.Adam(polynomial_model.parameters(), lr=lr)
for epoch in range(num_epochs):
for batch_idx in range(num_batches):
optim.zero_grad()
y_hat = polynomial_model.forward(X[batch_idx*batch_size:(batch_idx+1)*batch_size])
loss = torch.nn.functional.mse_loss(y_hat.cpu(), Y[batch_idx*batch_size:(batch_idx+1)*batch_size].cpu(), reduction='sum')
loss.backward(retain_graph=True)
optim.step()
print(f'epoch: {epoch}//loss:{loss.item():0.3f}')
print(f"Input Data equation is generated for the equation : {1} + {2}x + {3}X^2")
print(f"Equation after optimizing for {num_epochs*num_batches} iterations is : {polynomial_model.a.item()} + {polynomial_model.b.item()}x + {polynomial_model.c.item()}X^2")
epoch: 0//loss:2745.956
epoch: 1//loss:5327.916
epoch: 2//loss:1.913
epoch: 3//loss:0.983
epoch: 4//loss:0.944
epoch: 5//loss:3.251
epoch: 6//loss:8.481
epoch: 7//loss:3.731
epoch: 8//loss:0.562
epoch: 9//loss:0.484
epoch: 10//loss:0.741
epoch: 11//loss:0.556
epoch: 12//loss:0.304
epoch: 13//loss:0.153
epoch: 14//loss:0.070
epoch: 15//loss:0.028
epoch: 16//loss:0.008
epoch: 17//loss:0.001
epoch: 18//loss:0.000
epoch: 19//loss:0.001
epoch: 20//loss:0.002
epoch: 21//loss:0.002
epoch: 22//loss:0.002
epoch: 23//loss:0.002
epoch: 24//loss:0.001
Input Data equation is generated for the equation : 1 + 2x + 3X^2
Equation after optimizing for 1550 iterations is : 1.0060665607452393 + 1.9992820024490356x + 3.000042200088501X^2
Model variables
for param_tensor in polynomial_model.state_dict():
print(f"\t {param_tensor}: {polynomial_model.state_dict()[param_tensor]}")
a: 1.0060665607452393
b: 1.9992820024490356
c: 3.000042200088501
Optimizer variables
for param_tensor in optim.state_dict():
print(f"\t {param_tensor}: {optim.state_dict()[param_tensor]}")
state: {0: {'step': tensor(1550.), 'exp_avg': tensor(0.0296, device='cuda:0'), 'exp_avg_sq': tensor(28273.8398, device='cuda:0')}, 1: {'step': tensor(1550.), 'exp_avg': tensor(0.6155, device='cuda:0'), 'exp_avg_sq': tensor(1985039.1250, device='cuda:0')}, 2: {'step': tensor(1550.), 'exp_avg': tensor(8.0943, device='cuda:0'), 'exp_avg_sq': tensor(1.5650e+08, device='cuda:0')}}
param_groups: [{'lr': 0.1, 'betas': (0.9, 0.999), 'eps': 1e-08, 'weight_decay': 0, 'amsgrad': False, 'maximize': False, 'foreach': None, 'capturable': False, 'differentiable': False, 'fused': False, 'params': [0, 1, 2]}]
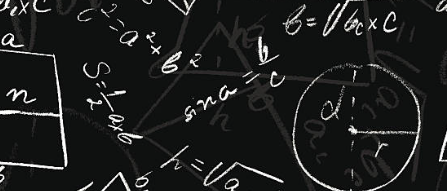